DEEP NEURAL NETWORK FOR CLICK-THROUGH RATE PREDICTION
DOI:
https://doi.org/10.15282/ijsecs.8.2.2022.4.0101Keywords:
Click-Through Rate, Deep Neural Network, Preprocessing, Advertising, Social NetworkAbstract
Predicted clickthrough rate is one of the most frequently used criteria to determine the effectiveness of an ad. In advertising production, click-through predictions are very influential for the company that places the ad. In addition to predicting the click-through rate of an ad, the use of the model or algorithm used is also very important in analyzing the click-through rate that occurs. The purpose of this study is to compare two advertising and social network datasets, by proposing the application of the Deep Neural Network (DNN) model by testing hyperparameter variations to find a better architecture in predicting click-through rates. The hyperparameter variations include 3 variations of the hidden layer, 2 variations of the activation function, namely ReLu and Sigmoid, 3 variations of the optimizer (RMSprop, Adam, and Adagrad) and 3 variations of the learning rate (0.1, 0.01, and 0.001). Experiments conducted with the advertising parameter dataset with hidden layer 3, learning rate 0.01 and Adam optimization resulted in the highest model with an accuracy value of 99.90%, AUC 99.90% and Precision-Recall 99.89%, while the social network ads parameter data with hidden layer 5, learning rate 0.1 and Adam optimization resulted in the highest model with an accuracy value of 92.25%, AUC 92.72% and Precision-Recall 89.70%.
References
T. BYRNES, “Advertising,” [Online]. Available: https://www.kaggle.com/datasets/tbyrnes/advertising.
“Social Network Ads,” [Online]. Available: https://www.kaggle.com/datasets/sash1563/social-network-ads.
Y. Xie, D. Jiang, X. Wang, and R. Xu, “Robust transfer integrated locally kernel embedding for click-through
rate prediction,” Inf. Sci. (Ny)., vol. 491, pp. 190–203, 2019, doi: 10.1016/j.ins.2019.04.006.
D. Jiang, R. Xu, X. Xu, and Y. Xie, “Multi-view feature transfer for click-through rate prediction,” Inf. Sci. (Ny).,
vol. 546, pp. 961–976, 2021, doi: 10.1016/j.ins.2020.09.005.
P. Saha, “Performance analysis of the Machine Learning Classifiers to predict the behaviour of the customers, when a new product is launched in the market,” vol. 5, no. 3, pp. 1907–1911, 2019.
S. Saraswathi, V. Krishnamurthy, D. Venkata Vara Prasad, R. K. Tarun, S. Abhinav, and D. Rushitaa, “Machine
learning based ad-click prediction system,” Int. J. Eng. Adv. Technol., vol. 8, no. 6, pp. 3646–3648, 2019, doi:
35940/ijeat.F9366.088619.
J. Mahindra Bagul and T. B. Kute, “Ad-Click Prediction using Prediction Algorithm: Machine Learning
Approach,” Int. Res. J. Eng. Technol., 2020, [Online]. Available: www.irjet.net.
A. Shrestha and A. Mahmood, “Review of deep learning algorithms and architectures,” IEEE Access, vol. 7, pp.
–53065, 2019, doi: 10.1109/ACCESS.2019.2912200.
S. M. Ieee, F. Ieee, V. Sze, Y.-H. Chen, T.-J. Yang, and J. S. Emer, “Efficient Processing of Deep Neural
Networks: A Tutorial and,” Proc. IEEE, vol. 105, no. 12, pp. 2295–2329, 2017, [Online]. Available:
http://ieeexplore.ieee.org/document/8114708/.
A. Noviar and S. Mukti, “Klasifikasi Arritmia pada Sinyal EKG menggunakan Deep Neural Network,” JUPITER,
vol. 13, pp. 29–38, 2021.
C. Y. Low, J. Park, and A. B. J. Teoh, “Stacking-Based Deep Neural Network: Deep Analytic Network for Pattern
Classification,” IEEE Trans. Cybern., vol. 50, no. 12, pp. 5021–5034, 2020, doi: 10.1109/TCYB.2019.2908387.
G. Li, F. Hu, Y. Zhao, and N. Chi, “Enhanced performance of a phosphorescent white LED CAP 64QAM VLC
system utilizing deep neural network (DNN) post equalization,” 2019 IEEE/CIC Int. Conf. Commun. China,
ICCC 2019, no. Iccc, pp. 173–176, 2019, doi: 10.1109/ICCChina.2019.8855926.
D. Xianzhi, “Research on Camera Calibration Technology Based on Deep Neural Network in Mine
Environment,” Proc. - 2020 Int. Conf. Comput. Vision, Image Deep Learn. CVIDL 2020, no. Cvidl, pp. 375–379,
, doi: 10.1109/CVIDL51233.2020.00-68.
B. LAILIAH, “AD CLICK PREDICTION DENGAN MACHINE LEARNING,” Tesis, 2021.
T. G.S., S. Dheeshjith, S. S. Iyengar, N. R. Sunitha, and P. Badrinath, “A hybrid and effective learning approach
for Click Fraud detection,” Mach. Learn. with Appl., vol. 3, p. 100016, 2021, doi: 10.1016/j.mlwa.2020.100016.
T. Çakmak, A. T. Tekin, Ç. Şenel, T. Çoban, Z. E. Uran, and C. Okan Sakar, “Accurate prediction of
advertisement clicks based on impression and click-through rate using extreme gradient boosting,” ICPRAM 2019
- Proc. 8th Int. Conf. Pattern Recognit. Appl. Methods, no. Icpram, pp. 621–629, 2019, doi:
5220/0007394306210629.
H. Sabita, F. Fitria, and R. Herwanto, “Analisa Dan Prediksi Iklan Lowongan Kerja Palsu Dengan Metode Natural
Language Programing Dan Machine Learning,” J. Inform., vol. 21, no. 1, pp. 14–22, 2021, doi:
30873/ji.v21i1.2865.
Oscar, N. Maulidiah, A. Purnamawati, D. Putri, and H. F. Pardede, “Prediksi Tingkat Kesuksesan Promosi Bank
Dengan Algoritma DNN.” 2021.
T. Çakmak, A. T. Tekin, Ç. Şenel, T. Çoban, Z. E. Uran, and C. Okan Sakar, “Accurate prediction of
advertisement clicks based on impression and click-through rate using extreme gradient boosting,” ICPRAM 2019
- Proc. 8th Int. Conf. Pattern Recognit. Appl. Methods, no. February, pp. 621–629, 2019, doi:
5220/0007394306210629.
D. Gunawan, “Evaluasi Performa Pemecahan Database dengan Metode Klasifikasi Pada Data Preprocessing Data
mining,” Khazanah Inform. J. Ilmu Komput. dan Inform., vol. 2, no. 1, p. 10, 2016, doi: 10.23917/khif.v2i1.1749.
M. T. Leleuly and P. H. Gunawan, “Analysis of Feature Correlation for Music Genre Classification,” 2020 8th
Int. Conf. Inf. Commun. Technol. ICoICT 2020, pp. 5–8, 2020, doi: 10.1109/ICoICT49345.2020.9166333.
J. K. Kim and S. Kang, “Neural Network-Based Coronary Heart Disease Risk Prediction Using Feature
Correlation Analysis,” J. Healthc. Eng., vol. 2017, 2017, doi: 10.1155/2017/2780501.
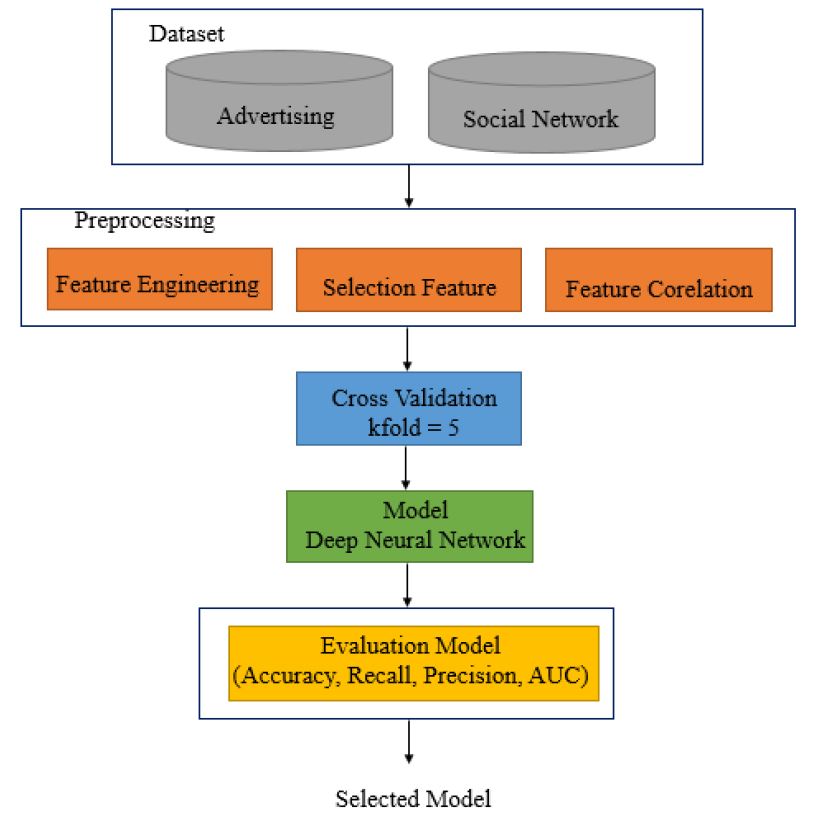
Downloads
Published
Issue
Section
License
Copyright (c) 2022 ERNI, DWIZA RIANA

This work is licensed under a Creative Commons Attribution-NonCommercial 4.0 International License.