OPTIMAL RESOURCE SCHEDULING ALGORITHM FOR OFDMA-BASED MULTICAST TRAFFIC DELIVERY OVER WIMAX NETWORKS USING PARTICLE SWARM OPTIMIZATION
DOI:
https://doi.org/10.15282/ijsecs.7.2.2021.6.0089Keywords:
Simulated Kalman filter, Opposition-based learning, Current optimumAbstract
Researchers are yet to entirely mapped out the difficulty in allocating optimal resources to mobile Worldwide Interoperability for Microwave Access (WiMAX) subscribers. This research presents an optimal scheduling algorithm for WiMAX resource allocation based on an Particle Swarm Optimization (PSO). In this work, sub-group creation is used to offer a PSO-based technique for allocating subcarriers and Orthogonal Frequency Division Multiplexing (OFDM) symbols to mobile WiMAX customers. The WiMAX network environment is organized into seven layers, with seven different modulation and coding algorithms proposed for sending packets to subscribers within each layer. By adopting an improved PSO-based WiMAX resource allocation method, an enhanced model for throughput maximization and channel data rate was implemented. The Aggregate Data Rate (ADR) and Channel Data Rate (CDR) for each scenario were obtained by simulating several scenarios of WiMAX multicast service to mobile users. Based on the performance evaluation of the enhanced algorithm for ADR and CDR, the results for the various layers and uniform distribution of users over the full layers were 350Mbps, 525Mbps, 700Mbps, 1050Mbps, 1050Mbps, 1400Mbps, 1575Mbps, and 1398Mbps. 6.98Mbps, 10.48Mbps, 13.97Mbps, 20.95Mbps, 20.95Mbps, 27.94Mbps, 31.5Mbps, and 28Mbps were also achieved for CDR. The significance of optimal resource allocation is to achieved a maximum ADR and CDR. The results showed a fair distribution of resources within the coverage area of the network .
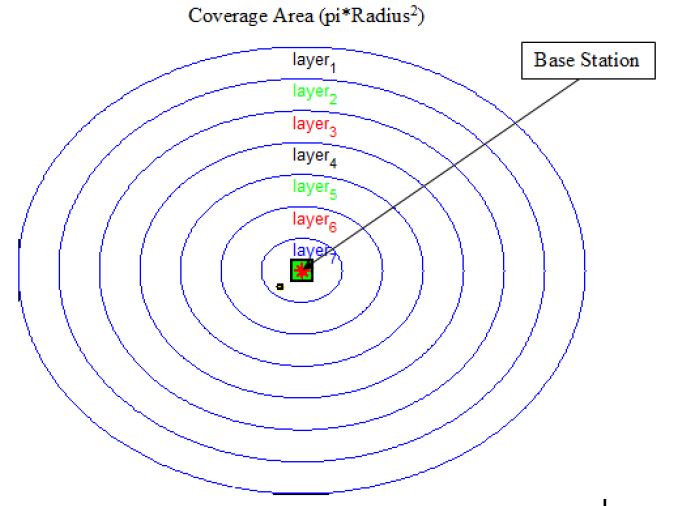