REDESIGNING POST-OPERATIVE PROCESSES USING DATA MINING CLASSIFICATION TECHNIQUES
DOI:
https://doi.org/10.15282/ijsecs.7.2.2021.7.0090Keywords:
Data mining, Data modelling and simulation, Decision making, Neural networks, Bayes’ networks, Healthcare managementAbstract
Data mining classification models are developed and investigated in this paper. These models are adopted to develop and redesign several business processes based on post-operative data. Post-operative data were collected and used via the Waikato Environment for Knowledge Analysis (WEKA), to investigate the factors influencing patients’ admission after surgery and compare the developed DM classification models. The results reveal that each implemented DM technique entails different attributes affecting patients’ post-surgery admission status. The comparison suggests that neural networks outperform other classification techniques. Further, the optimal number of beds required to accommodate post-operative patients is investigated. The simulation was conducted using queuing theory software to compute the expected number of beds required to achieve zero waiting time. The results indicate that the number of beds required to accommodate post-surgery patients waiting in the queue is the length of 1, which means that one bed will be available due to patient discharge.
References
I. Witten, E. Frank, and M. Hall, Data Mining: Practical Machine Learning Tools and Techniques, 4th ed.
Cambridge, USA : Morgan Kaufmann, pp, 2016, p. 655.
M. Hammer and J. Champy, Reengineering the Corporation: Manifesto for Business Revolution. London: Brealey
Pub, 2009, p. 272.
J. Han, M. Kamber, and J. Pei, Data Mining: Concepts and Techniques, Amsterdam: Elsevier/Morgan Kaufmann,
, p. 744.
S. C. Pandey, "Data mining techniques for medical data: A review," 2016 International Conference on Signal
Processing, Communication, Power and Embedded System (SCOPES), 2016, pp. 972-982.
W. Fan and A. Bifet. “Mining big data,” ACM SIGKDD Explorations Newsletter, vol. 14, no. 2, pp. 1–5, 2013.
M. Silver, T. Sakata, H. C. Su, C. Herman, M. J. O’Shea, and S. B. Dolins, “Case study: How to apply data mining
techniques in a healthcare data warehouse,” J. Healthc. Manag., vol. 15, no. 2, pp. 155–164, 2001.
N. Jothis, N. A. A. Rashid, and W. Husain, “Data mining in healthcare – A review,” Procedia Comput. Sci., vol.
, pp. 306–313, 2015.
M. J. Zaki and W. Meira, Data Mining and Analysis: Fundamental Concepts and Algorithms, New York, NY,
USA: Cambridge Univ. Press, 2017.
O. Folorunso and A. Ogunde, “Data mining as a technique for knowledge management in business process
redesign,” Information Management & Computer Security, vol. 13, no. 4, pp.274–280, 2005.
M. Islam, M. Hasan, X. Wang, H. Germack, and M. Noor-E-Alam, “A Systematic Review on Healthcare
Analytics: Application and Theoretical Perspective of Data Mining,” Healthcare, vol. 6, no. 2, p. 54, May 2018.
M. Persson and J. Persson, "Analysing management policies for operating room planning using
simulation", Health Care Management Science, vol. 13, no. 2, pp. 182-191, 2010.
K. Kasikumar, M. MohamedNajumuddeen and R. Suresh, “Applications of Data Mining Techniques in
Healthcare and Prediction of Heart Attacks.” International Journal of Data Mining Techniques and
Applications vol 7, pp.172-176, 2018.
M. Islam, M. Hasan, X. Wang, H. Germack and M. Noor-E-Alam, "A Systematic Review on Healthcare
Analytics: Application and Theoretical Perspective of Data Mining", Healthcare, vol. 6, no. 2, p. 54, 2018.
P. Ahmad, S. Qamar and S. Qasim Afser Rizvi, "Techniques of Data Mining In Healthcare: A
Review", International Journal of Computer Applications, vol. 120, no. 15, pp. 38-50, 2015.
P. Murphy and D. Aha, "UCI Machine Learning Repository", 1992. [Online]. Available:
http://archive.ics.uci.edu/ml. [Accessed: 23- Aug- 2020].
J. Bell, Machine Learning: Hands-On For Developers And Technical Professionals, 2nd ed. Wily, 2020.
J. David, "Data Mining and Data Warehousing", The SIJ Transactions on Computer Science Engineering & its
Applications (CSEA), pp. 17-19, 2019.
A. Segatori, F. Marcelloni and W. Pedrycz, "On Distributed Fuzzy Decision Trees for Big Data," in IEEE
Transactions on Fuzzy Systems, vol. 26, no. 1, pp. 174-192, Feb. 2018.
A. I. Georgevici and M. Terblanche, “Neural networks and deep learning: a brief introduction,” Intensive Care
Medicine, vol. 45, no. 5, pp. 712-714, Feb. 2019.
O. Akgobek, “A Rule Induction Algorithm for Knowledge Discovery and Classification,” Turkish Journal of
Electrical Engineering & Computer Sciences, vol. 21, no. 5, pp. 1223-1241, 2013.
J. Cheng and R. Greiner, "Comparing Bayesian Network Classifiers." UAI'99: Proceedings of the Fifteenth
conference on Uncertainty in artificial intelligence, Alberta, 1999, pp. 101–108.
R. Pi, Y. Cai, Y. Li and Y. Cao, "Machine Learning Based on Bayes Networks to Predict the Cascading Failure
Propagation," in IEEE Access, vol. 6, pp. 44815-44823, 2018.
S. Kilic, "Chi-square Test", Journal of Mood Disorders, vol. 6, no. 3, p. 180, 2016.
A. Richardson, "Nonparametric Statistics: A Step-by-Step Approach", International Statistical Review, vol. 83,
no. 1, pp. 163-164, 2015.
G. Zacharewicz, "Generalized Discrete Event System specification: A state-of-the-art study", Simulation, 2018.
E. Cepeda, "Stochastic coalescence multi-fragmentation processes", Stochastic Processes and their Applications,
vol. 126, no. 2, pp. 360-391, 2016.
K. Balakrishnan, Exponential Distribution: Theory, Methods and Applications, London: Routledge, 2020.
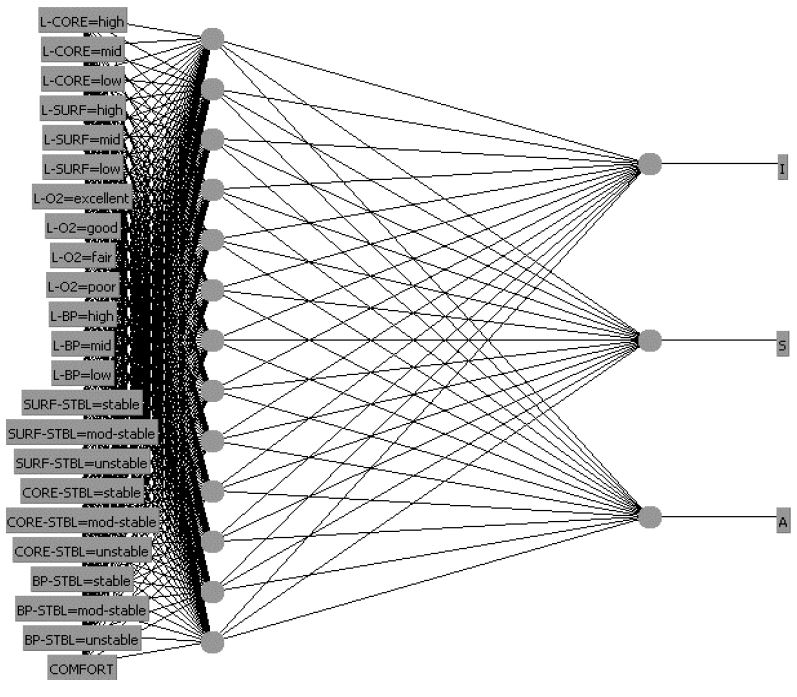
Downloads
Published
Issue
Section
License
Copyright (c) 2021 Hayder Ghazi Alwattar

This work is licensed under a Creative Commons Attribution-NonCommercial 4.0 International License.