A COMPUTER-AIDED DIAGNOSIS (CAD) SYSTEM FOR AUTOMATIC COUNTING OF KI67 CELLS IN MENINGIOMA
DOI:
https://doi.org/10.15282/ijsecs.8.2.2022.2.0099Keywords:
Automated Counting, Computer-Aided Diagnosis, Ki67, Meningioma, Tumour GradingAbstract
Meningioma is a type of primary brain tumour where this tumour arises in the three thin layers of tissues, called meninges. Tumour grading is usually used to describe tumour cells' characteristics and behaviours and how they look under a microscope. There were many techniques used for determining the grade of the tumour. Ki67 was the most common proliferation marker used to measure cell proliferation activity. Currently, pathologists used the manual counting technique to count the Ki67 cells before determining tumour grading. However, this technique was time-consuming, tiring and the counting results are often not accurate. Besides that, manual counting has poor reproducibility and discordant between counting values’ among the pathologist. Therefore, this study aimed to develop a Computer-Aided Design (CAD) software that automatically counts the Ki67 cells for determining tumour grading. The purpose of developing this software is to alleviate pathologists’ workload associated with counting Ki67 cells and scoring the Ki67 index. The CAD software was developed through seven stages. Based on Pearson Correlation Coefficient results, there was a good positive correlation between the proposed technique with the manual counting technique in counting positive and negative Ki67 cells with a correlation of 0.99 and 0.72 respectively. The proposed CAD system also showed promising results in computing the Ki67 labeling index with a low percentage absolute error of 1.85%.
References
American Cancer Society, “Cancer Facts & Figures 2017 - 2019,” Am. Cancer Soc., pp. 1–40, 2017.
Q. T. Ostrom, H. Gittleman, G. Truitt, A. Boscia, C. Kruchko, and J. S. Barnholtz-Sloan, “CBTRUS statistical report: Primary
brain and other central nervous system tumors diagnosed in the United States in 2011-2015,” Neuro. Oncol., vol. 20, pp. 1–
, 2018.
L. T. Li, G. Jiang, Q. Chen, and J. N. Zheng, “Ki67 is a promising molecular target in the diagnosis of cancer (Review),” Mol.
Med. Rep., vol. 11, no. 3, pp. 1566–1572, 2015.
J. H. Neltner, H. Su, and F. Xing, “Comparison of Manual and Automatic Methods of Ki-67 Proliferation Index For
Neuroendocrine Tumors: The Development and Validation of a Novel Digital Pathology Tool (Ki67 Counter),” 11th Annu.
ENETS Conf., vol. 43, no. 3, pp. 250–250, 2014.
C. G. Loukas, G. D. Wilson, B. Vojnovic, and A. Linney, “An image analysis-based approach for automated counting of
cancer cell nuclei in tissue sections,” Cytometry, vol. 55A, no. 1, pp. 30–42, 2003.
A. Aslantaş, E. Dandil, S. Saǧlam, and M. Çakiroǧlu, “CADBOSS: A computer-aided diagnosis system for whole-body bone
scintigraphy scans,” J. Cancer Res. Ther., vol. 12, no. 2, pp. 787–792, 2016.
C. A. Schneider, W. S. Rasband, and K. W. Eliceiri, “NIH Image to ImageJ: 25 years of image analysis,” Nat. Methods, vol.
, no. 7, pp. 671–675, 2012.
M. D. Abràmofff, P. J. Magalhães, and S. J. Ram, “Image processing with ImageJ Part II,” Biophotonics Int., vol. 11, no. 7,
pp. 36–43, 2005.
V. Tuominen, S. Ruotoistenmäki, A. Viitanen, M. Jumppanen, and J. Isola, “ImmunoRatio: a publicly available web
application for quantitative image analysis of estrogen receptor (ER), progesterone receptor (PR), and Ki-67,” Breast Cancer
Res., vol. 12, no. 4, p. R56, 2010.
S. Robertson, H. Azizpour, K. Smith, and J. Hartman, “Digital image analysis in breast pathology—from image processing
techniques to artificial intelligence,” Transl. Res. J. Lab. Clin. Med., vol. 194, pp. 19–35, 2018.
Ö. Demir and A. Y. Çamurcu, “Computer-aided detection of lung nodules using outer surface features,” Biomed. Mater. Eng.,
vol. 26, pp. S1213–S1222, 2015.
D. Ştefănescu, C. Streba, E. T. Cârţână, A. Săftoiu, G. Gruionu, and L. G. Gruionu, “Computer aided diagnosis for confocal
laser endomicroscopy in advanced colorectal adenocarcinoma,” PLoS One, vol. 11, no. 5, pp. 1–9, 2016.
A. M. Karim, F. V. Çelebi, and A. S. Mohammed, “Software Development for Blood Disease Expert System,” Lect. Notes
Softw. Eng., vol. 4, no. 3, pp. 179–183, 2016.
N. A. Shaharuddin and W. M. H. W. Mahmud, “Development of computer aided diagnosis system (CAD) for detection of
kidney ultrasound images,” 2017 Int. Conf. Eng. Technol. Technopreneurship, ICE2T 2017, no. September, pp. 1–4, 2017.
M. H. Bahreyni-Tossi, M. S. Moghadam, and S. Nekooie, “A NEW CAD SOFTWARE FOR EVALUATION OF BREAST
LESIONS IN CONTRAST – ENHANCED MR MAMMOGRAPHY,” Int. J. Life Sci. Pharma Res., vol. 8, no. 2, pp. 2–7,
X. Li, H. Yang, H. Huang, and T. Zhu, “CELLCOUNTER: Novel open-source software for counting cell migration and
invasion in vitro,” Biomed Res. Int., vol. 2014, no. Figure 1, 2014.
J. O’brien, H. Hayder, and C. Peng, “Automated quantification and analysis of cell counting procedures using imagej plugins,”
J. Vis. Exp., vol. 2016, no. 117, 2016.
R. Gonz�lez-Gonz�lez et al., “Comparison between Manual and Automated Methods for Ki-67 Immunoexpression
Quantification in Ameloblastomas,” Anal. Cell. Pathol., 2016.
A. Loddo, L. Putzu, C. DI Ruberto, and G. Fenu, “A Computer-Aided System for Differential Count from Peripheral Blood
Cell Images,” Proc. - 12th Int. Conf. Signal Image Technol. Internet-Based Syst. SITIS 2016, no. November 2017, pp. 112–
, 2017.
C. Venter and C. U. Niesler, “Rapid quantification of cellular proliferation and migration using ImageJ,” Biotechniques, vol.
, no. 2, pp. 99–102, 2019.
American Cancer Society, “Testing Biopsy and Cytology Specimens for Cancer,” Am. Cancer Soc., p. 20, 2015.
J. Kaur and A. Choudhary, “Comparison of Several Contrast Stretching Techniques on Acute Leukemia Images,” Int. J. Eng.
Innov. Technol., vol. 2, no. 1, pp. 332–335, 2012.
N. Otsu, “A Threshold Selection Method from Gray-Level Histograms,” IEEE Trans. Syst. Man. Cybern., vol. 20, no. 1, pp.
–66, 1979.
Khushbu and I. Vats, “Otsu Image Segmentation Algorithm:,” Int. J. Innov. Res. Comput. Commun. Eng., vol. 5, no. 6, pp.
–11948, 2017.
R. C. Gonzalez and R. E. Woods, Digital Image Processing, 3rd ed. New Jersey, USA: Pearson Education, 2008.
L. Vincent, “Morphological Area Openings and Closings for Grey-scale Images,” in Shape in Picture, Berlin, Heidelberg:
Springer, 1994.
G. Kumar and P. K. Bhatia, “A detailed review of feature extraction in image processing systems,” in International Conference
on Advanced Computing and Communication Technologies, ACCT, 2014, pp. 5–12.
D. N. Louis et al., “The 2016 World Health Organization Classification of Tumors of the Central Nervous System: a
summary,” Acta Neuropathol, vol. 131, no. 6, pp. 803–820, 2016.
S. T.Smith, MATLAB: Advanced GUI Development, 1st ed. Indianapolis: Dog Ear Publishing, 2006.
P. Purkait, B. Biswas, S. Das, and C. Koley, “Measurement of Errors,” in Electrical and Electronics Measurements and
Instrumentation, New Delhi: McGraw-Hill Education, 2013.
Y. Zhang, H. Wang, Z. Yang, and J. Li, “Relative Accuracy Evaluation,” PLoS One, vol. 9, no. 8, pp. 1–13, 2014.
S. Jimson, L. Malathi, G. M. K. Kumar, and N. Balachander, “Artifact in histological section,” Biomed. Pharmacol. J., vol.
, no. 2, pp. 843–845, 2016
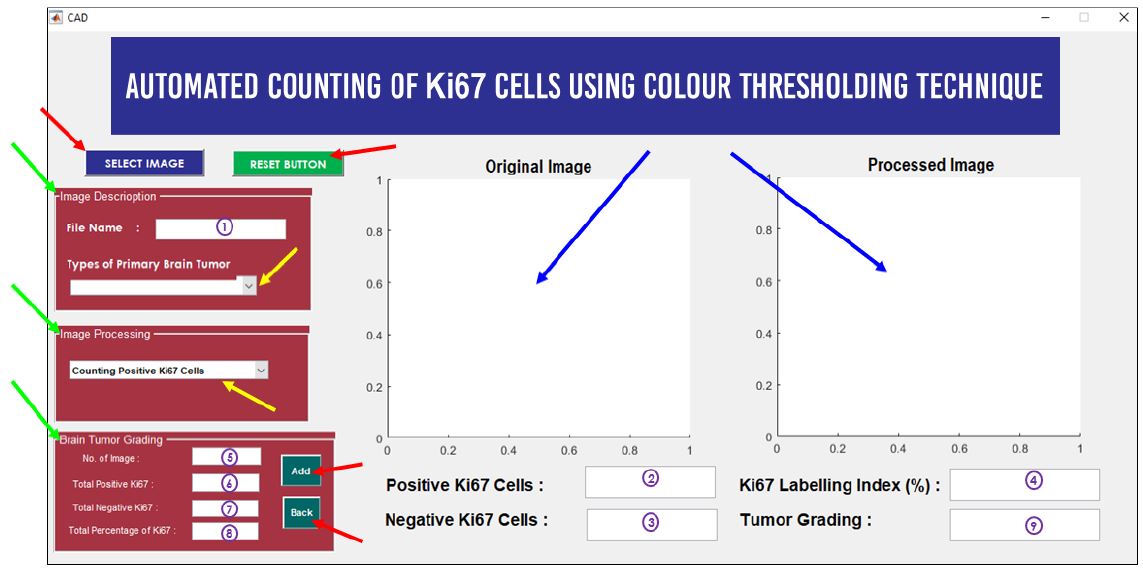
Downloads
Published
Issue
Section
License
Copyright (c) 2022 FAHMI AKMAL DZULKIFLI, MOHD YUSOFF MASHOR, HASNAN JAAFAR

This work is licensed under a Creative Commons Attribution-NonCommercial 4.0 International License.